By Sailesh Adhikari
Activity-based costing (ABC) model is a method of assigning indirect costs to products and services (Rappold, 2006). It was first introduced in the late 1970s by the Consortium for Advanced Management- International (CAM-I) (Quesada, 2010). ABC accounting model was designed to be applicable to any kind of organization regardless of product kinds, production method, and level of automation (Andersch, et.al 2014). Kaplan and Burns (1987) describe ABC model as a more appropriate method to allocate increasing overhead cost due to advancement in technology and reduced labor-intensive work, compared to traditional costing method. Because of this, the model is widely and variously used.
ABC model is applied by obtaining the cost of each activity required to develop or produce the final product and assigning the share of the costs to unit volume of the product based on all processing activities. The first step in activity-based costing involves identifying activities and classifying them according to the cost hierarchy. Cost hierarchy is a framework that classifies activities based on the ease with which they are traceable to a product (Ainsworth et al, 2003). To allocate the costing in a process, traditional costing components are divided into four different levels as unit-level costs, batch-level costs, product-level costs, and facility-level costs (Lere 2000). This allows high fixed overhead costs to be allocated to specific activities that occur in the manufacturing process (Rappold, 2006). Unit level activities are activities that are performed on each unit of product. Batch-level activities are activities that are performed whenever a batch of the product is produced. Product-level activities are activities that are carried out separately for each product. Facility-level activities are activities that are carried out at the plant level. The unit-level activities are most easily traceable to products while facility-level activities are least traceable (Rappold, 2006).
The main advantage of the ABC method over traditional methods is the ability to recognize different costing activity as measures of value addition instead of only considering volume or quantity of the product as in traditional costing practice (Rappold, 2006). This recognition of different costing activities helps to distribute the fixed cost evenly to each product output. The major limitation of implementing ABC costing model in the real field is the time and knowledge of the process and model (Lere 2000).
Howard (1993) developed an equation to determine the variable cost of processing individual logs into lumber which required that a variable cost function for each machine center be calculated based upon the labor costs, maintenance costs, and utility costs incurred at each machine center (Rappold, 2006). The equation proposed by Howard (1993) to determine the costing of lumber from softwood logs is:

Where,
LVC = total variable cost for log “i”
PTIj = processing time for log “i” at machine center “j”
MCj = variable costs per scheduled hour for machine center “j”
m= number of machine centers with processing time function in Group 1, used to process all or part of the log “i”
n = total number of machine centers used to process all or part of the log “i”
n – m = number of machine centers with processing time functions in Group 2, used to process all or part of the log “i”
On this same model Howard (1993) defines the machines groups as follows; if the processing times of individual boards or logs can be measured for activities from any machine, they are the Group 1 machines and if it is not possible to measure the processing times for individual product for activities from any machine are Group 2 machines. The variable costs of the Group 1 machine centers are measured as a function of the individual pieces. The variable costs of the Group 2 machine centers are measured as a function of volume (Howard 1993). Howard’s equation acknowledges that not all machine centers are uniformly utilized when processing logs.
According to Garrison et. al., (1999), to implement the ABC model there may be different approach but the six core steps of costing are the major which are identified as:
Step 1: Identified activities are grouped together in activity pools
Step 2: Analysis activity identifies indirect cost and assigns it to an end product
Step 3: Based on the findings of step-1 and step-2, assign a cost to an activity pool
Step 4: Calculate activity rates for final product
Step 5: Assign the cost to cost objects with reference to identified activity pools and rates
Step 6: Prepare the costing reports
Application of ABC model to determine the hardwood and softwood CLT cost
The cost of the CLT can be evaluated based on ABC model, as discuss earlier, to determine the variable cost of CLT production. The ABC model is more appropriate in this context because there will be two different products from same process that vary only in primary raw materials and each process with different raw material (may) have different functioning factor and time. To implement the ABC model, six core steps of costing implementation, as discussed by (Garrison et. Al., 1999), will be followed. The overall process of the cost evaluation is presented in Figure 1.
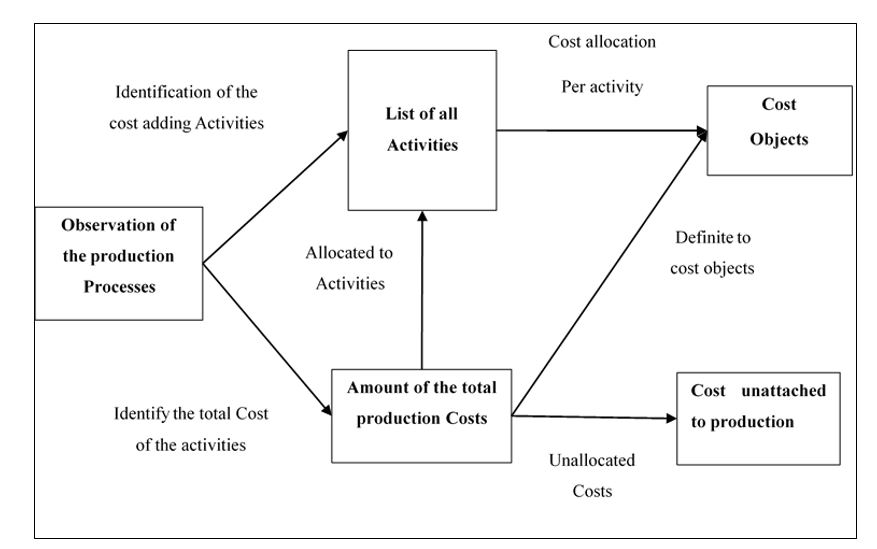
Figure 1: Purposed ABC model for product costing of CLTs.
For the cost analysis of the CLT production, all the activity that adds economic value to the product will be identified and grouped together in activity pools. The activity pools can be studied as Unit level, Batch Level, and Product Level. The overall possible activities in the process of CLT manufacturing include but not limited to:
CLT system design cost
Raw material acquisition cost
- Direct material cost
- Purchase order cost
- Delivery cost
- CLT processing cost
- Direct labor cost
- Machine setups
Operational cost
- Primary planning and QC check cost
- Finger jointing cost
- Board cutting
- Adhesive application cost
- Pressing and drying cost
- Trimming and edging cost
- Quality test cost
- CNC Processing for the architectural plans
- Product packaging cost
- Machine testing and calibration cost
- Maintenance and cleaning cost
- Transportation cost
- Installation of the CLT system
- Management cost
- Administrative cost
- Advertisement cost
- Insurance/ software cost
In the second step, the activity analysis will be performed to identify total indirect costs for manufacturing CLT from both softwood and hardwood (SPF/SYP and yellow poplar). Those costs will be allocated to an end product. In the third step, the cost is allocated to an activity pool. Considering the cost of each activity pool, activity rates for the final product are calculated in the fourth step. Once activity costs, pools, and rates are identified and clearly defined, the next step is to allocate cost to cost objects. With all the information obtained, the financial report will be prepared in the final step. The total cost of the CLT will be the sum of the cost from production to the installation of the CLT system.
References:
- Ainsworth, P., & Deines, D. McGraw-Hill Higher Education New Product Listing: Titles due for publication April-June 2003 BUSINESS.
- Andersch, Adrienn; Buehlmann, Urs; Palmer, Jeff; Wiedenbeck, Janice K.; Lawser, Steve. 2014. Product costing guide for wood dimension and component manufacturers. Gen. Tech. Rep. NRS-140. Newtown Square, PA: U.S. Department of Agriculture, Forest Service, Northern Research Station. 31 p
- Garrison, Ray H., and Eric W. Noreen. Managerial Accounting. 9th ed. Boston: Irwin McGraw-Hill, 1999.
- Howard, A. F. (1993). A method for determining the cost of manufacturing individual logs into lumber. Forest products journal, 43(1), 67.
- Kaplan, Robert S., and W. Bruns. 1987. Accounting and Management: A Field Study Perspective. Boston: Harvard Business Publishing
- Lere, J.C. 2000. Activity-based costing: A powerful tool for pricing. J. Bus. Ind. Mark. 15(1):23–33.
- Quesada, H. P. (2010). The ABCs of Cost Allocation in the Wood Products Industry: Applications in the Furniture Industry. Blacksburg: College of Agriculture and Life Sciences, Virginia Polytechnic Institute and State University, PUBLICATION 420-147.
- Rappold, P.M. 2006.Activity-based product costing in a hardwood sawmill through the use of discrete-event simulation. Ph.D. dissertation, Virginia Polytechnic Inst. and State Univ., Blacksburg, Virginia. Available at: http://scholar.lib.vt.edu/theses/available/etd-06122006-162052/. 250 pp